FOR DATA ANALYSIS
AI / ML Operations
We provide a human-in-the-loop model to solve highly complex data labelling requirements and deliver 99.9% quality training data for your advanced machine-learning projects.
Key facts
OBJECTS
IMAGES
%
ACCURACY
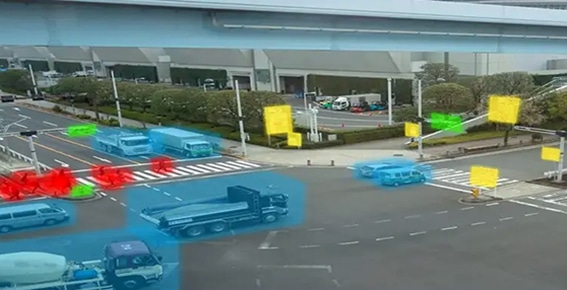
Image
Annotation
Image annotation is the practice of labelling images to train AI and machine learning models. It is similar to data labelling but in the context of visual data. Annotated data assists AI/ML models to recognize, detect and discriminate objects from each other. Annova offers expert annotation delivering the best results.
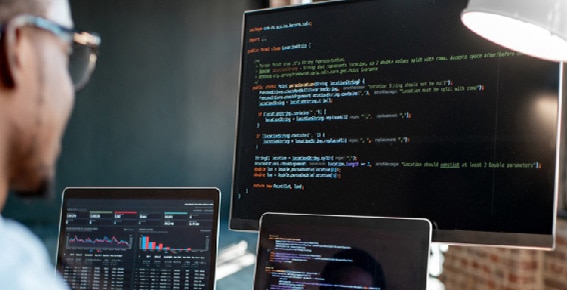
Text
Annotation
Text annotation identifies and labels sentences with metadata to define the characteristics of sentences enabling MLs modules to understand human conversations better. Poorly annotated data can deliver irrelevant or misleading responses. At Annova we follow meticulous text labeling to ensure no information is overlooked.
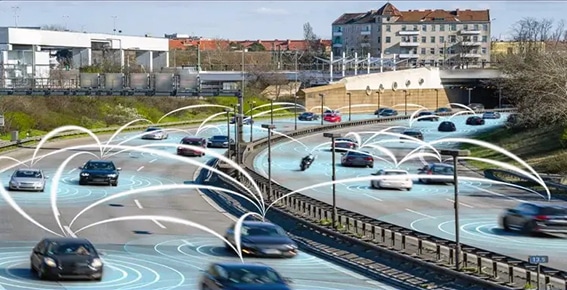
Video
Annotation
Video annotation assists AI-powered algorithms in identifying objects in videos. Precisely annotated videos create a high-quality reference database that can be used by computer vision-enabled systems to accurately identify objects such as cars, people, and animals. Partner with Annova to experience 99.9% accuracy.
Segments We Cover
NLP
Annova’s NLP service offers a comprehensive suite of tools and techniques to unlock valuable insights from textual data. Leverage cutting-edge technology to drive efficiency and innovation across a wide range of applications.
Named Entity Recognition (NER)
Named Entity Recognition (NER) is a subtask of natural language processing (NLP) that involves identifying and classifying named entities in text. Named entities are words or phrases that refer to specific types of entities, such as people, organizations, locations, or dates. NER algorithms use machine learning techniques, such as deep neural networks, to identify and extract these entities from text, and they can be further categorized into predefined classes based on their type. Entity classification is the process of assigning a category or label to an entity based on its type or characteristics. Together, NER and entity classification can be used in a wide range of applications, such as information retrieval, question answering, and sentiment analysis.
Sentiment Analysis
Sentiment analysis is a natural language processing technique that involves identifying and extracting the emotional tone or attitude expressed in text. It is often used in applications such as social media monitoring, customer feedback analysis, and brand reputation management. Sentiment analysis algorithms typically use machine learning techniques to analyze large amounts of text data and classify it as positive, negative, or neutral based on the language used and the context in which it appears. Topic analysis, on the other hand, is a text-mining technique that involves identifying the main themes or topics present in a document or collection of documents. It is often used in applications such as content categorization, information retrieval, and trend analysis. Topic analysis algorithms use statistical methods, such as latent semantic analysis, to identify patterns in the text data and group related words and phrases together based on their semantic similarities.
Audio and Text Transcription
Audio and text transcription are techniques used to convert spoken or recorded speech into written text. Audio transcription involves listening to an audio recording and transcribing it word-for-word into a written document, while text transcription involves converting written text from one language to another. Transcription can be done manually or through the use of automated speech recognition software. Automated transcription uses machine learning algorithms to analyze the audio or text data and convert it into written form. Audio and text transcription are widely used in applications such as captioning videos, creating transcripts of meetings or interviews, and creating subtitles for movies or television shows. They are also useful in enabling accessibility for people with hearing impairments or those who speak different languages.
Intent and Conversation Analysis
Intent and conversation analysis are techniques used in natural language processing to extract meaning from spoken or written language. Intent analysis involves identifying the purpose or goal behind a user’s request, while conversation analysis involves analyzing the structure and flow of a conversation to determine its effectiveness and identify areas for improvement. Intent analysis algorithms typically use machine learning techniques to analyze user input and classify it into one or more predefined categories based on the user’s intent. This technique is often used in applications such as chatbots, virtual assistants, and customer service interactions. Conversation analysis algorithms, on the other hand, use natural language processing and machine learning techniques to analyze the structure and content of a conversation and identify patterns and trends in the data. This technique can be used to identify areas where conversations are breaking down or where users are becoming frustrated or confused, enabling companies to improve their customer service and engagement.
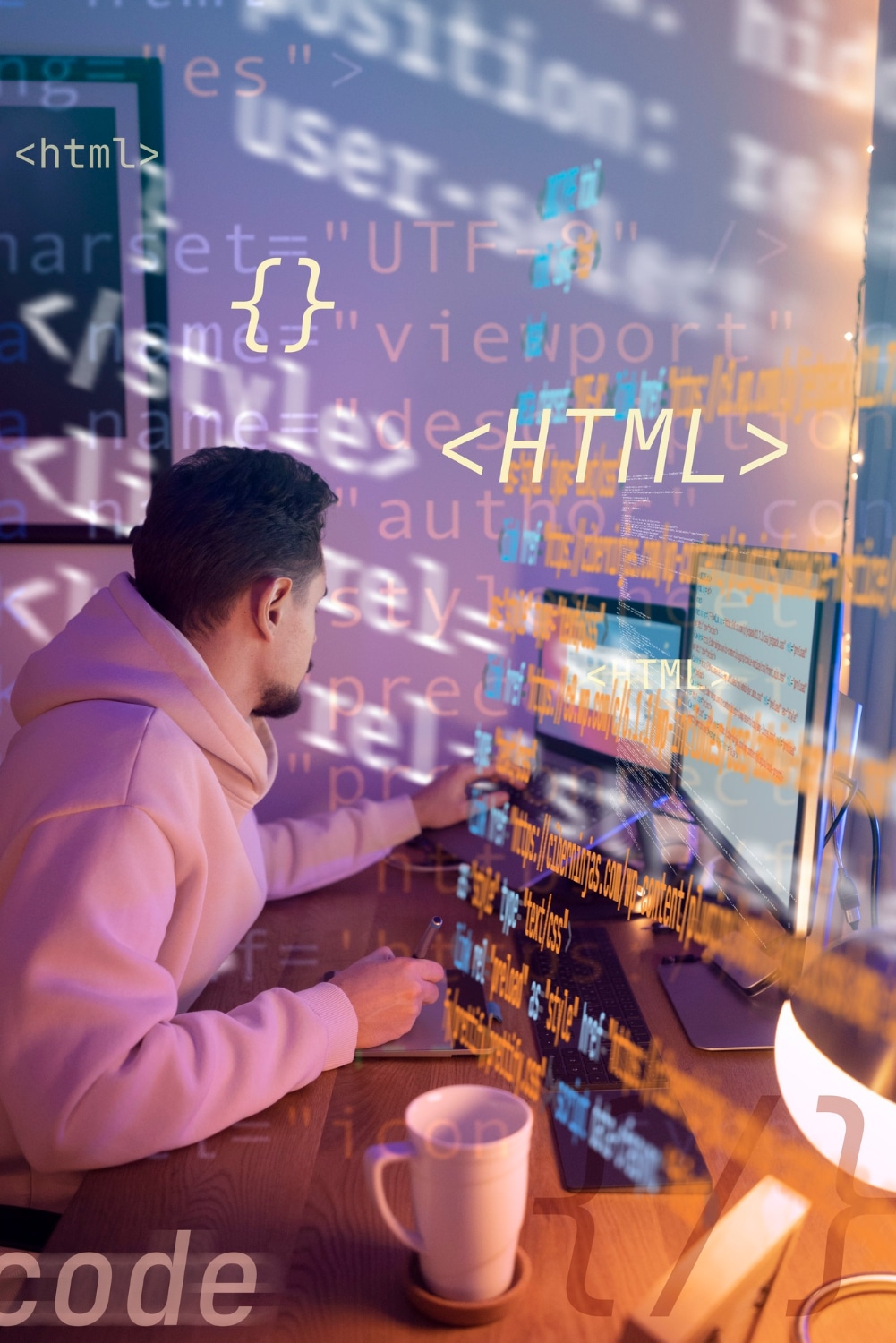
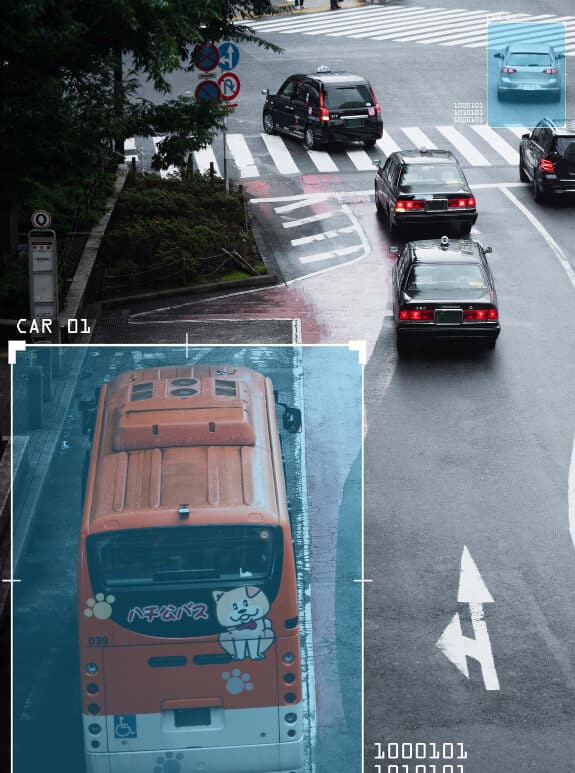
Computer vision
With highly accurate training data annotation across major computer vision tasks, Annova enables the development of world-class AI perception models for various industries. Our expertise spans all data types and our rigorous quality control ensures precision-annotated datasets.
Bounding Boxes
Bounding boxes or rotating bounding boxes are one of the most popular image annotation techniques in deep learning. A bounding box is an imaginary rectangle that serves as a point of reference for object detection and creates a collision box for that object in projects on image processing. Data annotators draw these rectangles over machine-learning images, outlining the object of interest within each image by defining its X and Y coordinates. This makes it easier for machine learning algorithms to find what they’re looking for, determine collision paths, and conserve valuable computing resources.
Polygon Annotation
Polygon annotation is the process of object annotation by selecting a series of x, y coordinates along its edges to make annotation precise and accurate. It provides pixel-perfect precision, is highly flexible, and is adaptable to a variety of complex shapes. In this real-world environment where everything is dynamic, expecting regular-shaped data is not possible. However, annotating regular-shaped data is relatively easy as you need to bind it with a rectangular/ square box. Polygon annotation is used for irregular-shaped or simple, images which you can’t bind inbox. Polygon Annotation captures more lines and angles to draw a high level of accuracy. To best represent an object’s actual shape, annotators click at specific points to plot vertices and have the freedom to change direction whenever necessary.
Semantic Segmentation
Semantic segmentation is a computer vision technique that involves dividing an image into regions and assigning a label to each region based on its visual characteristics. Unlike object detection or classification, which focus on identifying specific objects within an image, semantic segmentation provides a more fine-grained understanding of the image’s contents by labeling every pixel according to the category it belongs to. This technique is particularly useful in applications such as autonomous driving, where precise identification of objects and their surroundings is crucial.
Panoptic Segmentation
Panoptic segmentation is a computer vision technique that aims to combine the strengths of both semantic segmentation and instance segmentation. It involves dividing an image into regions and assigning a label to each region based on its visual characteristics, while also identifying individual instances of objects within the image. The resulting output includes both pixel-level semantic labels and instance-level object masks, providing a more comprehensive understanding of the image’s contents. This technique is particularly useful in applications where it is important to distinguish between different instances of the same object, such as in crowded scenes or when tracking moving objects.
Lidar Annotation
Under Data Annotation and Data Labeling services one of the most important annotations for autonomous vehicles is LiDAR annotation.Inaccurate or incomplete annotation scans lead to vehicle decision-making errors, potentially resulting in accidents or other hazards. For this reason, Lidar annotation must be performed by experienced and trained professionals using top-notch tools and techniques. One of the challenges in Lidar annotation is the vast amount of data that must be processed. Lidar sensors can generate massive amounts of data, and manually annotating this data can be time-consuming and labour-intensive. This is where machine learning comes into play. By using machine learning algorithms, it is possible to automate and accelerate the annotation process while maintaining a high accuracy level.
Annova Solutions Pvt. Ltd.
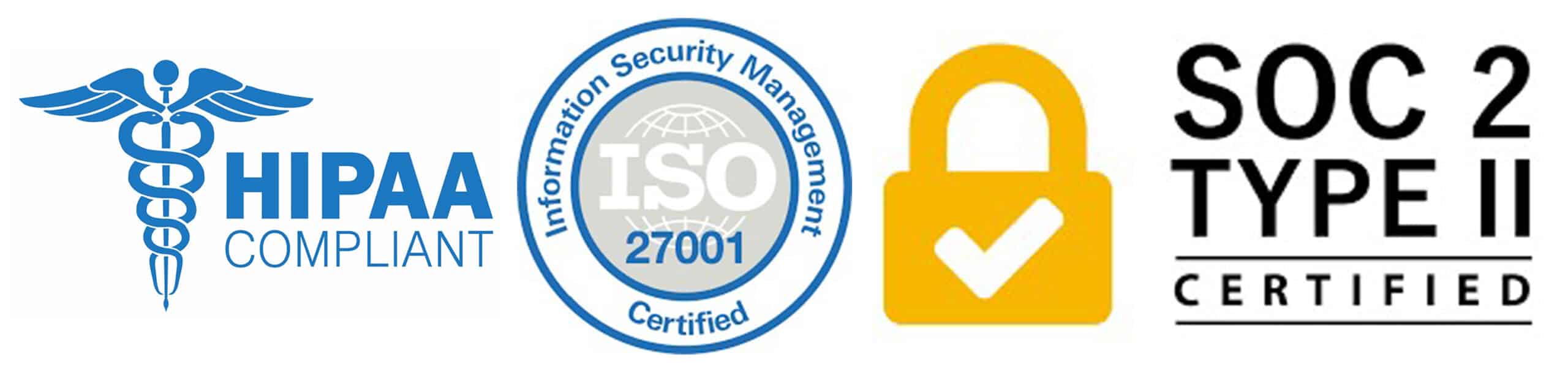
Quick Links
Our services
About us
Contact
Our services
Healthcare
AI/ ML Operations
Digital BPO